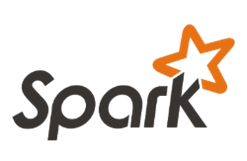
Apache Spark
Apache Spark is an open-source distributed computing system that provides a unified analytics engine for big data processing. It includes built-in libraries for machine learning (MLlib) and graph processing (GraphX), making it a powerful tool for large-scale data analysis and AI-driven applications.